Executive summary
We explored whether Medicare Advantage (MA) plans might influence traditional Medicare fee-for-service (FFS) costs. We determined that a strong relationship exists between higher (lower) MA market penetration rates and lower (higher) FFS trends. Based on this, we developed for consideration a modest adjustment to the Medicare Payment Advisory Commission's (MedPAC's) reported MA payment to FFS cost ratio.
The adjusted ratios indicate that estimated 2015 MA plan payment rates are slightly lower than FFS costs might be, absent significant MA market penetration. While the adjusted ratio is modestly different from MedPAC's ratios, we believe it presents a reasonable alternative to the MedPAC ratio often used to assess MA value.
Figure 1: Average differential between MA Plan Payments and FFS Cost
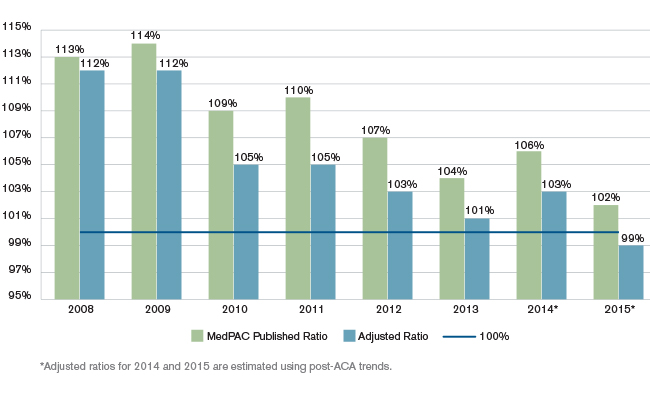
The adjusted ratio uses FFS costs restated, to remove the estimated impact MA plans appear to have on FFS costs in place of the FFS costs used in MedPAC's reported ratios.
Ultimately, it is near impossible to prove or disprove that MA market penetration causes change in FFS costs, as those costs are influenced by many factors. However, the strong statistical evidence that MA market penetration predicts FFS cost trends (p-value < 0.001) leads us to believe the adjusted ratio warrants consideration.
We provide more details below.
Study Overview
MedPAC's annual report estimates ratio of MA plan payments to Medicare FFS costs
In March of every year, MedPAC's report to Congress provides various traditional Medicare and MA cost analyses, including one comparing MA payments to costs for traditional Medicare members. For the past 10 years, MedPAC reported payments to MA plans were higher than traditional Medicare (or FFS) costs. Notably, the gap between MA plan payments and FFS costs narrowed since the Patient Protection and Affordable Care Act (ACA) reduced MA benchmark payment rates and, in turn, payments to MA plans. MedPAC estimates the MA plan payments were, on average, 102% of FFS spending in 2015.1 Figure 1 above includes the historical average ratio of MA plan payments to FFS costs, as reported by MedPAC.
MA enrollment grew from 5.6 million in 2005, prior to the implementation of Medicare Part D (PD) and the Centers for Medicare and Medicaid Services (CMS) MA-PD bidding process, to an estimated 16.8 million in 2015.2 This yielded a penetration rate increase (i.e., MA enrollment divided by total Medicare eligibles) from approximately 13% in 2005 to approximately 31% in 2015. In several counties, over half of the Medicare beneficiaries are currently enrolled in an MA plan.
MA program improvement efforts may also benefit Medicare FFS
In the MA program, plans strive to improve the quality and efficiency of care delivered to enrollees. For most plans under the current payment mechanism, such success is key to freeing up the financial resources to provide the additional benefits necessary to attract and retain enrollees while still appropriately contributing to operating margin. Typically, MA plans invest and work with their providers to realize quality and efficiency improvements sooner than would happen otherwise.
Because providers tell us they use the most appropriate approach for each of their patients (i.e., treatment doesn't differ depending on whether a Medicare beneficiary is in an MA plan or traditional Medicare), we believe MA plan improvement efforts are likely to cause a sentinel effect on Medicare FFS. In other words, we expect Medicare FFS costs will be favorably influenced by MA plan improvement efforts. If our premise holds, we would generally expect lower Medicare FFS cost trends, normalized for relative fee schedule changes, in counties with higher MA penetration rates, and higher trends in counties with lower MA penetration rates.
We investigated the relationship of MA penetration rates to Medicare FFS trends
We explored the potential impact of MA plan penetration rates on Medicare FFS cost trends, normalized for relative fee schedule changes, and found a strong relationship. The linear regression we performed on the county-level FFS cost trend and MA penetration rate data demonstrated strong statistical evidence (p < 0.001) that county MA penetration rate predicts cumulative FFS cost trend, normalized for relative fee schedule changes, from 2007 to 2013.
We included the estimated impact of MA penetration on FFS trends in a revised ratio
We adjusted MedPAC's reported MA plan payment to FFS cost ratios by excluding estimated trend savings related to the sentinel impact of MA plans. First, we grouped counties into cohorts based on their MA penetration rates:
- Minimal MA presence (counties with less than 3% MA penetration)
- Counties with MA penetration between 3% and 15%
- Counties with MA penetration between 15% and 23%
- Counties with MA penetration between 23% and 35%
- Counties with MA penetration over 35%
After selecting the minimal MA penetration cohort, we divided the remaining counties into approximate quartiles based on 2013 FFS enrollment. We used the minimal MA penetration cohort's cost trend (i.e., the baseline trend) to estimate cost trends without MA's presence and then calculated the difference between each cohort's trend and the baseline trend. The table in Figure 2 summarizes our estimates.
Figure 2: FFS Cost Trends at Various Medicare Advantage Penetration Levels
July 2013 MA Penetration* | 2013 FFS Enrollment | 2007 to 2013 Normalized Cost Trend | Difference From Normalized Baseline Trend |
Baseline |
|||
Less than 3% | 705,272 | 14.4% | 0.0% |
Significant MA Presence |
|||
3%-15% | 8,163,202 | 11.6% | -2.4% |
15%-23% | 8,010,557 | 11.7% | -2.4% |
23%-35% | 8,264,281 | 9.7% | -4.1% |
Over 35% | 8,352,332 | 7.6% | -5.9% |
Total/Composite | 33,495,644 | 10.2% | -3.7% |
*MA penetration levels only include members enrolled in pure MA plans, not Program of All-Inclusive Care for the Elderly (PACE), cost contracts, or demonstrations.
Figure 2 illustrates the strong statistical relationship we noted above between MA penetration rates and lower FFS cost trend. Normalized medical cost trends in counties with MA penetration greater than 35% were nearly 6% lower than counties with minimal MA penetration from 2007 to 2013. Nationwide, the composite cost trend was 3.7% lower than the baseline trend.
To develop the adjusted ratios in Figure 1 above, we increased the FFS costs used in MedPAC's ratios to add back the estimated cost reduction (i.e., the difference from baseline). The alternate ratios demonstrate what ratios might be if the potential sentinel effect on FFS costs resulting from the decreased cost trends attributed to relative MA plan presence is accounted for.
In Figure 1, we recognized the difference between the MedPAC and adjusted ratios decreases slightly from 2011 (110% vs. 105%) to 2015 (102% vs. 99%). Our analysis showed that average annual trends from 2011 to 2013, reflecting the ACA's impact starting in 2012, were significantly lower than the average trends over the prior four years. The table in Figure 3 summarizes these trends.
Figure 3: FFS Cost Trends Before and After ACA Implementation
Average Annual Trend | |||
July 2013 MA Penetration* | Before ACA (2007 to 2011) | After ACA (2011 to 2013) | Total |
Less than 3% (Baseline) | 3.5% | -0.1% | 2.3% |
3%-15% | 2.6% | 0.4% | 1.8% |
15%-23% | 2.7% | 0.3% | 1.9% |
23%-35% | 2.3% | 0.1% | 1.5% |
Over 35% | 1.8% | 0.1% | 1.2% |
Total | 2.3% | 0.2% | 1.6% |
* MA penetration levels only include members enrolled in pure MA plans, not Program of All-Inclusive Care for the Elderly (PACE), cost contracts, or demonstrations.
In addition, the average annual trends for the minimal MA presence cohort after the ACA was implemented were slightly lower than for the other quartiles. The opposite was true prior to the implementation of the ACA. Given this notable difference, we judged it appropriate to calculate the adjusted ratios for 2012 and 2013 using the post-ACA trends. Because we develop the adjusted ratio by restating all FFS costs using trends of only the minimal MA presence cohort, this modestly decreased the FFS costs used for the adjusted ratio for these two years. Therefore, the gap between the MedPAC ratio and the adjusted ratio in Figure 1 above narrows from 2011 through 2013.
For 2014 and 2015, we assumed the post-ACA FFS cost trends would continue when developing restated FFS cost estimates.
Data sources, methodology, and assumptions
Data sources
We estimated the potential MA cost-saving impact on FFS costs using publicly available databases provided annually by CMS. CMS releases per beneficiary FFS cost amounts and risk scores by county used to develop MA benchmark payment rates. We used the FFS cost amounts from these sources for 2007 to 2013, the most recent six years of data available, to develop the average annual trend for each of the previously mentioned cohorts. The FFS cost amounts were normalized using the provided risk scores to put all costs on a 1.00 risk score basis.
We developed FFS cost primarily using two CMS FFS cost files. Each FFS cost file contains five years of FFS cost and risk score data by county. CMS adjusted the FFS cost data in each of these files to put all counties on the same year of wage indices, Geographic Practice Cost Index (GPCI) factors, and other factors, thus avoiding changes in FFS costs caused by fee schedule changes, other than a nationwide inflation adjustment. We used the CMS file with FFS cost data from 2007 through 2011—the first data set CMS published with FFS costs adjusted for these fee schedule factors—for the first two years of trend, and the file containing data from 2009 through 2013 for the last four years of trend.
CMS also releases MA penetration rates each month, calculated as the percentage of Medicare beneficiaries in each county that are enrolled in an MA plan. We used a penetration rate based on pure MA plan enrollment only, thus ignoring enrollment in other programs such as PACE and Cost plans.
Methodology
We used the MA penetration rate data to group counties into cohorts based on their 2013 penetration rates. By using only the 2013 MA penetration rate and enrollment data, we eliminated the impact of county mix changes. We discuss sensitivity testing concerning the impact of MA penetration rate changes later in this report.
As part of our study, we adjusted the data as follows:
- We included only U.S. states and Washington, D.C., in our trend analysis.
- We excluded Maryland because of the difference in FFS payment methodology. Maryland was exempt from the Inpatient and Outpatient Prospective Payment Systems (IPPS and OPPS). As a result, CMS is unable to adjust the underlying inpatient and outpatient FFS cost data to be on a similar fee schedule base, thus making year-over-year trend comparisons difficult.
- We adjusted the FFS cost data for completion factors and sequestration, as appropriate.
Assumptions
We selected a cutoff of 3% MA penetration for the minimal MA presence cohort. The 3% threshold provides a point where trends represent large enough populations to be credible but also begin to show consistent patterns of reduced trends in relation to continued increasing MA penetration rates.
Another key assumption we made was in regards to the MedPAC calculation of the ratio of the MA plan payments to FFS costs, as shown in Figure 1 above. We assumed the numerator in the MedPAC ratio calculation (MA plan payment rates) would not change, which was due to our restatement of estimated FFS costs, because current law doesn't allow for such a restatement when determining MA benchmark payment rates. Beginning in 2012, MA plan payment rates could have been influenced by a change in FFS costs since the ACA began to require MA benchmark payment rates by county be calculated using some proportion of estimated FFS costs for a given county. However, the plan payment rates are ultimately based off CMS's estimate of FFS costs at the time of developing the benchmark payment rates.
In the course of our study, we considered numerous other factors that could influence FFS cost trends but the data available did not allow us to measure all of these factors independently and thus we could not draw conclusions on their estimated impacts.
Observations and considerations
We reviewed the following scenarios and/or assumptions and determined whether an adjustment to our analysis was necessary:
- We identified counties with MA penetration rate changes from 2009 to 2013 that varied meaningfully from the nationwide average increase of approximately 5%. We noticed counties with a significant increase in MA penetration during this time period had lower FFS cost trends than the nationwide average. Counties with a decrease in MA penetration during this time had higher FFS cost trends. The table in Figure 4 summarizes our observations.
Figure 4: FFS cost trends for counties with notable penetration rate changes
MA Penetration Change from 2009 to 2013 Membership Number of Counties 2009 to 2013 Trend >10% 3,115,763 359 3.7% < 0% 5,547,928 882 6.0% All Enrollees 33,495,644 3,112 4.3% The majority of the counties in the group with greater than 10% MA penetration growth are in the upper quartiles of this analysis, while counties with a decline in MA penetration are in the lower quartiles. Therefore, we didn't adjust our analysis for these shifts because these counties show similar findings to the overall result.
- In the 2017 Advance Notice, CMS proposed changes to the Hierarchical Condition Category (HCC) Risk Adjustment model for predicting costs of dual eligible beneficiaries. The HCC risk adjustment model is used to develop the risk scores provided in the CMS data files. The proposed changes would adjust risk scores for dual eligible beneficiaries to better align them with predicted cost. Revising the risk scores for all beneficiaries to be consistent with the Advance Notice could change the results of our analysis because we normalized the provided FFS costs using the provided risk scores. However, we reviewed the proportion of dual eligible beneficiaries and found their distribution within each cohort remained relatively steady over the analysis period. Therefore, we expect the impact of the HCC risk adjustment model changes would have minimal effect on the trends developed in this study.
- We reviewed the impact on our results if we excluded counties with relatively small or large FFS populations. Low-population counties tended to fall into lower MA penetration cohorts, while high-population counties tended to be grouped into high MA penetration cohorts. We noticed these counties, on average, exhibited trends that followed the trend pattern predicted by their MA penetrations (i.e., low-population counties have higher trend rates). As such, we did not exclude any counties for falling below or exceeding a population threshold.
- We also reviewed the impact of removing counties with trend outliers—those counties with trends more than 25% above or below the average six-year trend over all counties. We observed only minor changes in the predictability of MA penetration rate on trends after removing the outliers.
- While we selected a cutoff of 3% MA penetration rate for the minimal MA presence cohort, we also tested this assumption at 5% as well. This had the effect of increasing the number of members in the cohort from roughly 700,000 to approximately 1.8 million. We then redistributed the remaining membership to similar size quartiles. The composite trend difference between the minimal presence cohort and the average drops from -3.7% to -2.7% with the 5% threshold. However, the post-ACA trends in the 5% threshold scenario are near equal to the minimal MA presence cohort and the composite managed group. In total, the adjusted ratios in Figure 1 above increase by 1% in 2008, 2012, and 2013. The ratios increase by 2% in 2010 and 2011. All other Figure 1 ratios remain the same, including the 2015 adjusted ratio of 99%.
Our trend analysis showed trends begin to steadily decline around 3% MA penetration. Thus, increasing the cutoff point begins to introduce a fair amount of managed members into the unmanaged cohort. - Because our analysis estimated how much MA might influence FFS cost trends, we also considered the potential sentinel impact MA might have on FFS risk scores. In other words, we considered whether FFS risk scores might be overstated relative to what they would be absent MA, which would be due to MA plan efforts to improve provider diagnoses coding. We evaluated the sensitivity on our results if reported FFS risk scores are inflated because of MA penetration. Assuming reported FFS risk scores are inflated by 1% in a theoretical 100% MA penetration rate county, our testing indicates the composite trend differential is inflated by 0.1%. We scaled the 1% impact in a theoretical 100% MA penetration rate county by each county's MA penetration rate (i.e., for our 1% FFS risk score overstatement scenario, a 20% MA penetration rate county was modeled with a 0.2% FFS risk score overstatement). Thus, the 0.1% composite trend differential reflects the nationwide mix of county-specific penetration rates.
- We evaluated whether several other factors might influence our results but estimated little impact. These other factors included:
- CMS's readmission reduction program, which began to adjust payments to providers in 2013 for high inpatient (IP) readmission rates
- Trend variations for counties where provider-owned plans enroll a significant portion of the MA population
- Effect of MA competition within a county on trends, including:
- Number of plans available
- Size of largest competitor in county
Statistical relevance
We performed a linear regression analysis on the county-level FFS cost and penetration rate data, which was used as the basis for our study. The linear regression analysis showed a p-value of less than 0.001, which implies MA penetration rate is a good predictor of cost trend. The regression coefficient (slope) for all counties was -0.204, implying a 10% increase in the MA penetration rate reduces the six-year cost trend by 2.04%. We also performed a membership-weighted regression on the same data. The results of this analysis also yielded a p-value of less than 0.001 and a regression coefficient of -0.143.
In addition, we considered if variables other than penetration rate influence trend. Some of these factors (e.g., county size) likely influence where MA organizations establish plans, but it was important to determine if these other variables directly influence trend. We ran regression analyses on rural counties (membership-weighted regression coefficient = -0.050; 22% of total membership), urban counties (-0.128; 78%), all counties with more than 10,000 FFS members (-0.122; 72%), and only those counties with 25,000 or more FFS members (-0.118; 56%). While the rural factor was less dramatic but still meaningful, the other tests showed similar regression coefficients to the all-county analysis and all had p-values less than 0.001. Thus, within each of these groupings we noticed directionally similar relationships of penetration rate to trend as we did over all counties.
Conclusion
We explored whether the MA program has not only provided significant value to MA members but also helped reduce FFS cost trends through MA plans striving to improve the quality and efficiency of care delivered to enrollees. The results show MA penetration rates are a strong predictor of FFS cost trends. Thus, when comparing MA plan payments to FFS costs, consideration should be given for how MA plans might impact FFS costs.
It is important to note that it is near impossible to prove one factor was the sole driver of the change in trends. We did, though, attempt to analyze other measurable factors that could also influence FFS cost trends. There are additional factors that could influence these trends but the data available did not allow us to measure all of them independently, and thus we could not draw conclusions on their estimated impacts. We don't believe, however, that the results of our analysis would have changed significantly.
As we noted earlier, it is near impossible to prove or disprove that MA market penetration causes change in FFS costs, as those costs are influenced by many factors. Yet the strong statistical evidence that MA market penetration predicts FFS cost trends (p < 0.001) leads us to believe the adjusted ratio warrants consideration. Our numerous attempts to analyze other measurable factors that could influence FFS cost trends didn't yield results refuting our analyses. Unfortunately, significant challenges exist with data availability and the dependence of other measurable factors.
Caveats
In performing our analysis, we relied on data published by CMS. We did not audit or verify this data. If the underlying data is inaccurate or incomplete, the results of our analysis may likewise be inaccurate or incomplete.
Guidelines issued by the American Academy of Actuaries require actuaries to include their professional qualifications in all actuarial communications. Andrew Mueller is a member of the American Academy of Actuaries and meets the qualification standards for performing the analyses in this report.
The material in this report represents the opinion of the authors and is not necessarily representative of the views of Milliman. As such, Milliman is not advocating for, or endorsing, any specific views contained in this report related to the Medicare Advantage program.
1MedPAC (March 2015). Report to the Congress: Medicare Payment Policy, p. 325. Retrieved February 29, 2016, from http://www.medpac.gov/documents/reports/mar2015_entirereport_revised.pdf?sfvrsn=0
2Kaiser Family Foundation (June 30, 2015). Medicare Advantage 2015 Spotlight: Enrollment Market Update. Retrieved February 29, 2016, from http://kff.org/medicare/issue-brief/medicare-advantage-2015-spotlight-enrollment-market-update/