
The study was based on a data set containing around 2 million unique VA policies issued between 2003 and 2015 of seven large variable annuity writers based in the US. These policies represent roughly $220 billion of account value (based on initial purchase amounts) and cover a range of GLWB product designs as well as demographic attributes. This provides a rich data set with which to study policyholder behaviour.
A predictive model can be constructed with common variables such as age, tax-qualified status and single/joint status to allow easy implementation. The models constructed for our study use drivers that are readily available in a typical in-force data file, making them suitable for implementation in existing actuarial projection platforms. Including additional explanatory variables or interactions to the assumption formula is a natural step of predictive modelling because many variables can be captured in a single model without double-counting the individual variables' effects. This framework allows iterative improvements to predictions and better differentiation of policyholder behaviour at a seriatim level.
The 2016 Milliman VALUES GLWB Utilisation study examined both when the policyholders chose to begin taking lifetime withdrawals, as well as how efficiently they continued to take them thereafter. We were able to confirm and, more importantly, quantify many intuitive assumptions about these behaviours and what drives them, and discovered new insights as well. For example, less than half of all policyholders currently taking GLWB withdrawals utilise their GLWB benefit with 100% efficiency (i.e., taking precisely the maximum allowed withdrawal amount). This is interesting as we believe many companies price on a basis of 100% efficiency.
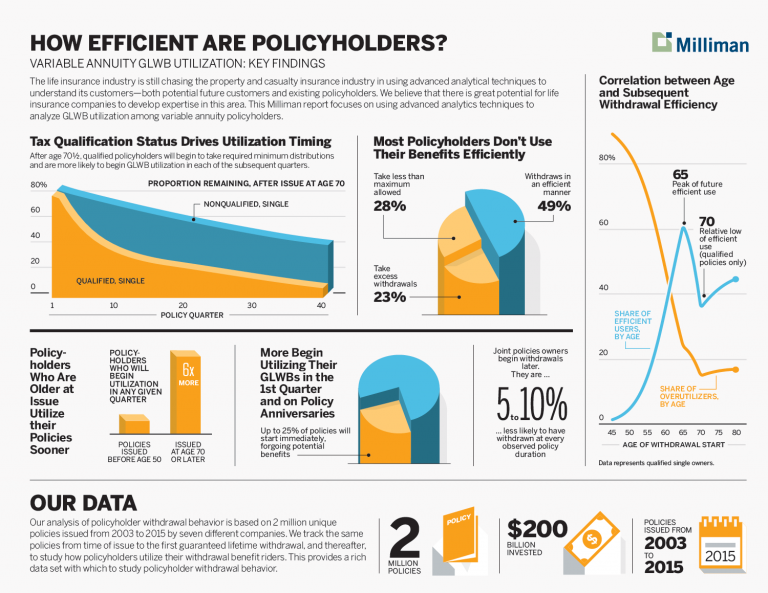
Other findings from our report include:
- Previous incidence of taking withdrawals (from their non-guaranteed funds) is a strong leading indicator of earlier GLWB utilisation
- Products with roll-up features tend to encourage later utilisation of GLWB
- Policyholders that utilise a lower proportion of their guarantee tend to be more likely to lapse
Predictive modelling of policyholder behaviour offers a statistically defensible framework for demonstrating assumption effectiveness to internal and external stakeholders. Rating agencies and regulators are placing higher scrutiny on how companies set assumptions around policyholder behaviour. A predictive model built on statistical principles provides sound validation metrics for measuring the effects of explanatory variables and the accuracy of the predictions. A broader application of predictive modelling is predicting variations in behaviour within a block of business under a range of stochastic scenarios to generate a distribution of behaviour outcomes. These types of analyses can be useful for demonstrating capital adequacy and strategic capital allocation.
For more information on the survey, read the 2016 Milliman VALUES GLWB Utilisation study . For more information on predictive modelling please contact your Milliman consultant.